Manasi Vartak‘s background includes positions and internships at Twitter, Google, and Facebook, and she is passionate about AI, ML and large scale data analytics. We caught up with her to find out more.
Tell us about your background leading up to founding Verta.
My journey in the tech industry goes back to my time at MIT, when I was working on my doctorate at CSAIL — the MIT Computer Science and Artificial Intelligence Laboratory. My thesis work evolved from my PhD project, which was called ModelDB. This was the first modern, open-source system for managing machine learning models. It was pioneering because, for the first time, it was addressing what model management should look like in machine learning and what capabilities a model management tool should include.
The initial versions of ModelDB were very closely informed by my experience prior to MIT. My background was in applied data science, working on projects like ad recommendation at Google, or analytics systems at Facebook. At Twitter I was working on their feed ranking system that shows you what tweets are most relevant to you. These experiences gave me a unique perspective on the needs of Data Science practitioners. I also developed the skills to be able to build tools to meet those needs.
What insights did you take away from your experience in industry about the challenges for Data Science today?
Every company today doing data science is trying to figure out how to meet the challenges around such issues as Generative AI, Large Language Models (LLMS), Responsible AI and AI Regulations. I was fortunate to work at some of the most AI-forward organisations, doing data science and machine learning at a larger scale and faster pace than most other organisations today. That experience gave me a very good perspective on the major challenges that data science teams face today. I’ve also benefited from working with our customers in the insurance industry to understand their pain points.
One fundamental challenge is model reproducibility. Data science, by definition, is very empirical. You’re doing a lot of experimentation. But typically none of that experimentation work gets tracked. When another data scientist in the same organization comes along and wants to solve that same problem, they have to start from scratch. So a lot of human time and a lot of compute resources get wasted during experimentation as you’re building and training your models.
On the production side, when that model gets put into a product and is running, the stakes are even higher. When a model is in production, if it’s not reproducible, that means that you can’t fix issues with it. Instead, you need to roll back the model to a version that works. Except, again, you have no idea which version that is or how it was generated, because none of that information has been tracked.
This issue of reproducibility is going to become even more acute as we see more regulations being put in place around artificial intelligence. A key requirement of proposed laws like the EU AI Act and the American Data Privacy and Protection Act is being able to report on which model was used to make a particular prediction, where the model came from, and what data it was trained on. Right now, it would be difficult or impossible for most organizations to pull that information together to comply with these regulations.
Two other challenges are around visibility and monitoring. First, with visibility, the problem is that companies simply don’t have a centralised way of tracking all their models, so they don’t know what models they have across the whole organisation and where they’re running. This is going to become a more serious problem when companies have to file reports with regulatory authorities on their entire portfolio. And finally, on monitoring, organisations haven’t had a way to track performance of their models consistently over time. These models might be impacting millions or even billions in revenue, but companies don’t have a view into how they’re performing and whether they’re generating value for the business.
Did your experiences inspire you to found Verta?
Working at some of the most AI-forward organisations, I came to see that, outside a handful of tech giants, most companies are still struggling to realise value from their data science investments. Lots of companies, including in the insurance sector, have invested heavily to build data science teams and enable those teams with tools. But they lack a system for effectively and efficiently operationalising models. It’s no surprise that we see analysts reporting that as many as 85% of models never make it into production.
I also believe that the future of AI is real-time, meaning models making real-time predictions in intelligent systems and products that are interacting directly with customers. In insurance, we see this with apps that let a customer file a claim with pictures of damage from their phone, and then it approves or denies the claim instantly, without any human intervention.
The implication of real-time is that companies are going to need their AI/ML applications to operate with the same extremely high reliability that we expect of other business-critical applications. Most companies simply aren’t prepared for that future, and they’ll be at a competitive disadvantage if they’re unable to apply the same level of rigor to delivering models as they do to other software that drives their business success.
So my inspiration to found Verta really came from a desire to bring the learnings that my colleagues and I took away from our experiences working at companies like Google, Twitter and NVIDIA, and enable any company to achieve the same kind of high-impact data science and machine learning that we had delivered in our past work at those AI leaders.
Tell us about Verta and what differentiates you from other market players.
In insurance, specifically, we have an established footprint in this industry, which, after all, is one of the original sectors where data science found broad application. We have proved to be a very good fit for insurers that have large-scale data science and that want to benefit from the latest infrastructure and advances in AI/ML, because we help tie all that together.
In general, AI/ML is a very diverse space, with varying levels of maturity in the technology to support AI/ML. Tools for developing, training and experimenting with models have been widely adopted, for example. But the tools for deploying, managing and monitoring models in production are at the early stages of adoption. Gartner has said this space is only 1-5% penetrated at this point, and companies are still using a lot of homegrown, one-off tools.
Verta’s unique focus and perspective on this space is that the way to see value from AI/ML and stay innovative is by focusing on the delivery and management side. The Verta Operational AI platform takes any ML model and instantaneously packages and delivers it using best-in-class DevOps support for CI/CD, operations, and monitoring, while ensuring safe, reliable, and scalable real-time AI deployments. Our Model Catalog provides centralized model inventory, robust model governance features, regulatory compliance tracking, and powerful model management capabilities.
Our differentiators include enterprise readiness, our ability to deploy into, and integrate with, diverse existing IT ecosystems, and to work seamlessly with any kind of model across any compute environment, whether on-prem, cloud, hybrid or multi-cloud. We can handle traditional batch workloads and also support real-time use cases at enterprise scale. We believe that these capabilities uniquely position Verta to deliver operational AI and enterprise model management at scale for a diverse set of Fortune 500 companies.
What is the company culture like at Verta?
Verta has a very diverse workforce, with women and people of colour in senior leadership positions, which is a strength of the organisation, bringing a wide set of perspectives to the team.
At the same time, we have a very high level of technical talent and operational expertise on the team, with practitioners who have deployed high-velocity operational AI systems at some of the largest AI-forward organisations. We have seen how best-practice ML is done at Fortune 500 companies.
When we work with our customers, not only are they getting a platform and system with these best practices baked in, but we are able to advise them on how to drive the greatest value from their ML. The fact that our team has walked a mile in our customers’ shoes gives us the knowledge and expertise to help them achieve best-in-class performance.
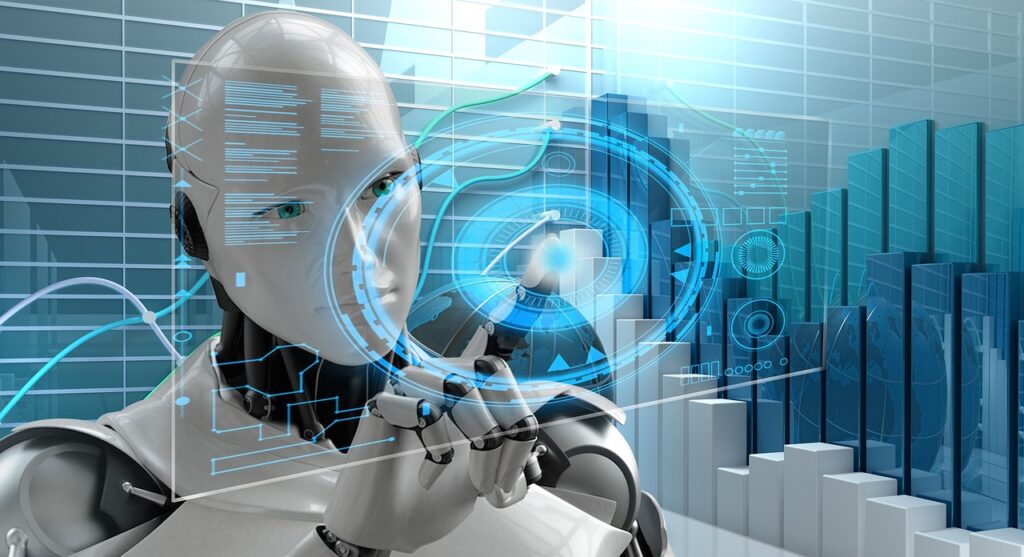
Generative AI has captured the public’s imagination. What opportunities and risks do you see for companies that want to leverage generative AI?
Generative AI is a really fascinating topic, and we do see both opportunities and risks associated with it, including in the insurance industry. On the opportunity side, it obviously has had a huge impact anywhere content creation is central. Marketing can use it to create more personalised content. Customer service can improve chatbots to deliver better information to clients. Software developers are generating code faster using generative AI.
However, we also see risks and challenges associated with using generative AI, both for insurance companies and organisations in other sectors. Insurance companies, for example, could see an increase in fraudulent claims that use images of accident damage created using generative AI. There are concerns around generative models producing biased outputs if the data they are trained on itself reflects biases. There are copyright issues still being worked out, too, since generative AI can be used to create near-identical copies of existing products or content. Output quality can also be a challenge, since these models are prone to produce “hallucinations” or factual errors.
This isn’t to say that companies shouldn’t be actively looking at how to use generative AI. But businesses need to carefully consider the implications of using the technology and take steps to mitigate potential risks.
What excites you about the future of this industry?
We’re on the cusp of an era when intelligent applications will touch every aspect of our lives. In insurance, we’ll see advances in functions like marketing, claims processing and customer service that are going to revolutionize the industry. It’s exciting to be leading a company that is poised to power this next generation of “smart” products and services. When you think about the impact that AI can have in applications that help individuals get faster relief from disasters, better service to solve their everyday challenges, and more security for their families – it’s a great opportunity to work with companies to positively impact how people live.
Join Verta in New York at ITI USA
Verta experts will be on hand at Insurtech Insights USA 2023, in New York, on June 7th and 8th at the Javits Center, to provide more information and strategies on their solutions and offerings. Come and see them on the expo floor – booth 205.
To find out more, click here